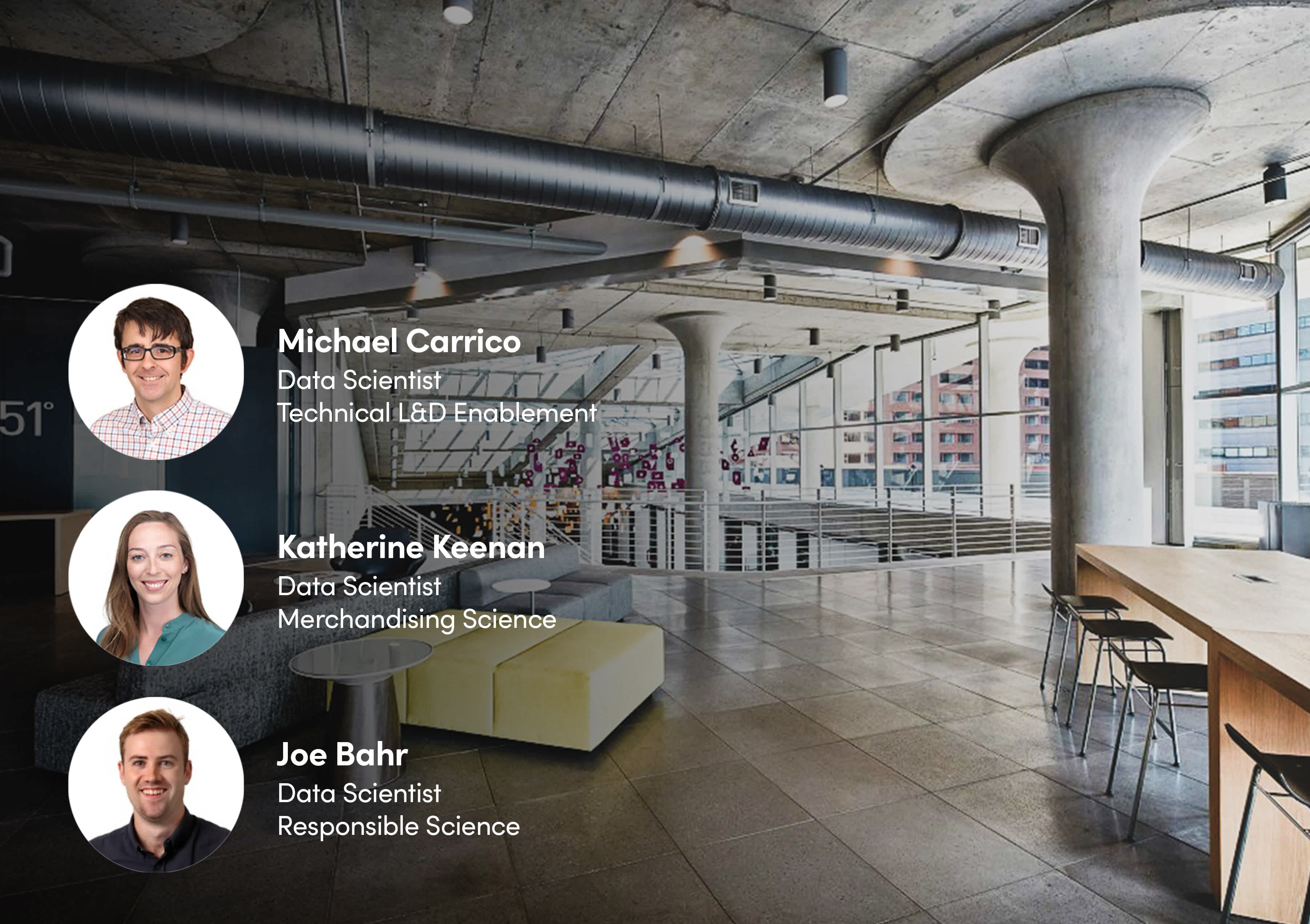
Gender bias in self-reported skills ratings
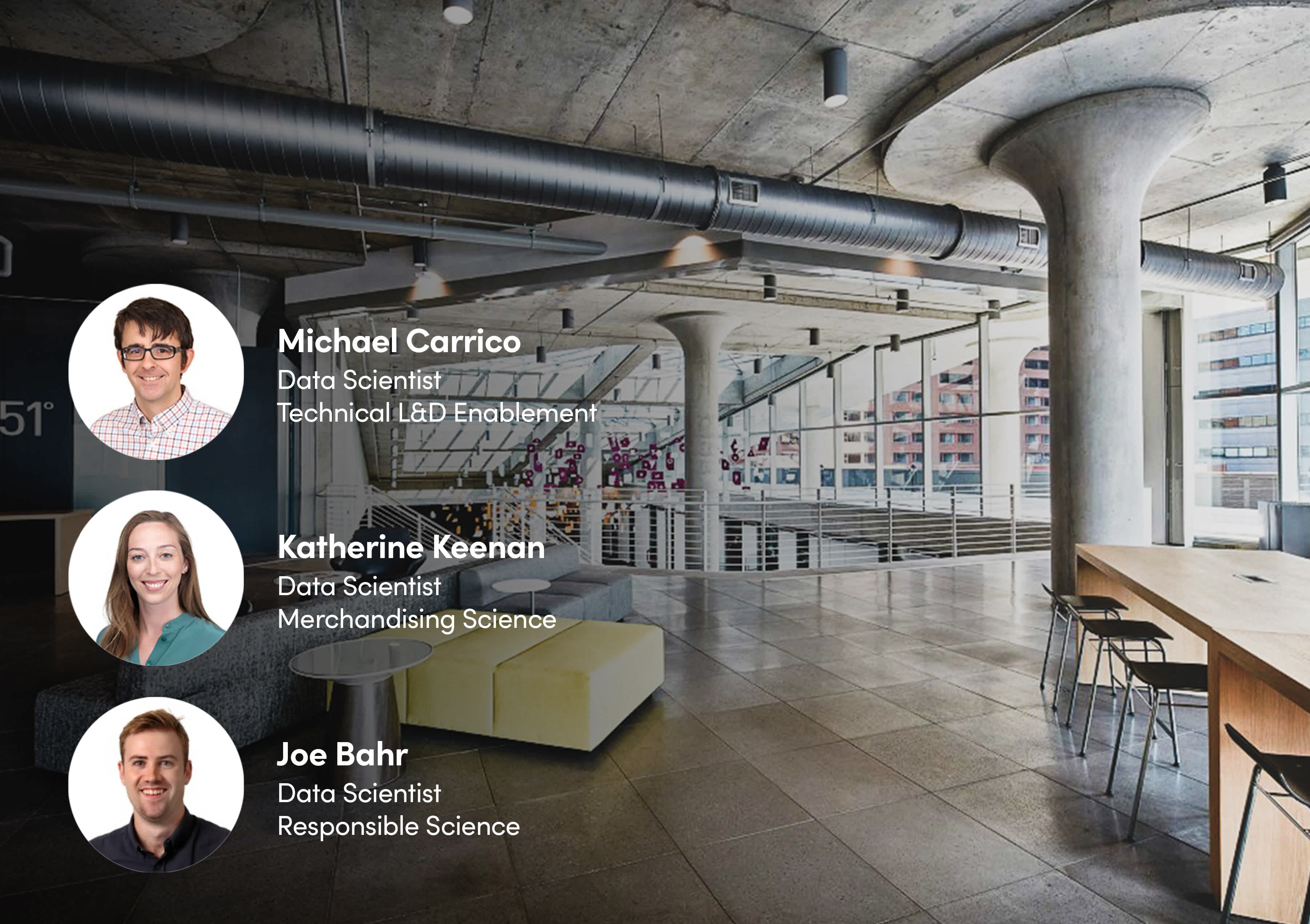
Welcome to 84.51° Data University, a series of quarterly insights for prospective and current data-science professionals.
Can you all give us some background on your work in mitigating gender bias within our organization?
We have made significant strides toward being a skills-based organization in influencing our talent strategies in everything from the roles we have, the trainings we need, talent mobility, etc. A big part of enabling that skills-based strategy involves exploring self-reported skills rating data.
Self-reported data has its own set of issues, but a big one we wanted to explore is gender disparity. Countless studies have shown that even when performance is equal, men rate theirs as 33% higher than women. We wanted to explore and action on that with our own data, so we conducted analyses and have implemented practices to ensure we have the right mitigation plans in place.
How did you all get started with this work?
A big shout out to Ilana Blumin and Rachel DaDamio as they did an initial analysis on gender bias in skills data through the Women in Tech group. Their work has inspired us to continue the practice even as our approach to skills has evolved and expanded.
“We have some grassroots as well as systematic plans in place to ensure women consider more technical roles and invest in technical skill development."
How do you think this work has helped us positively influence gender equity in skill development?
We are just scratching the surface, but I’d highlight three different areas today where we are already starting to see traction:
Data use. We are mindful of NOT tying performance decisions to self-rating data and are actively exploring how we can bring more objective rating systems to triangulate the data we have. We are also further educating our people on what a rating of X truly means so that our talent and people leaders can be more aligned and consistent.
Training content curation. When engaging trainers to help develop content, we are seeking more diverse perspectives in those groups. We are proactively seeking out under-represented groups across various facets of diversity to ensure that our training isn’t just developed for and by a small group of like-minded and similarly skilled individuals.
Talent pipelines. This data is also helping us identify more diverse populations for tech track and leadership positions. We have some grassroots as well as systematic plans in place to ensure women consider more technical roles and invest in technical skill development.
How do you think your skills and experiences as data scientists help with this more broadly?
Our entire organization is on the skills-based talent strategy journey. Having data scientists at the helm helps us ensure that we don’t make harmful decisions with data. We can also proactively share and reapply our data-based learnings and methods to other spaces.
Visit our knowledge hub
See what you can learn from our latest posts.