
Emily Gibbons talks AI and data development at Analytics Unite

A growing number of tech-savvy retailers and CPGs are strategically investing in data-powered solutions as they look to not only predict future demand, but realign their operations for maximum efficiency. Emily Gibbons, VP, Product & Technology, recently joined an expert panel discussion at the 2024 Analytics Unite Summit in Chicago to explore some of the findings in the newly released 2024 RIS and CGT Analytics Study, which benchmarks analytics maturity among select CPG and retail executives. Here are five key takeaways she shared during the session:
1. Start small and grow your analytic acumen
A lot of CPGs and retailers are feeling like they’re not doing enough in terms of their analytics resources, or that they’re far behind. I would encourage organizations to start putting data at the forefront of more problems. It is easy to be ‘wowed’ by the promises of complex and sophisticated science, but there is also an opportunity cost to doing nothing. Starting small helps to drive early value, while also giving a testing ground to ensure that, as you bring in sophistication, you do so with more knowledge and a clearly framed problem. Using data is a journey for everybody. The most important thing is to get started so you can build the analytic acumen of your organization.
2. Context is key in driving value from analytics
There's a lot in the study on how to structure your analytics team – centralized, federated to departments, within IT, etc. With so many models out there, it is important to think through the approach that makes the most sense for your business. Centralizing your team drives more scale – enabling you to manage best practices, governance, tooling, and the right platforms to enable your data scientists and your technology teams to do what they do best. But as you drive towards scalability, you can’t forget that context is key. Analytics is as much an art as it is a science, and it is important not to pull your analytic talent too far away from the business lines that they aim to serve. It's important to balance where you need scalability and where you need that frontline context to make sure that what’s being deployed by those teams is relevant and solving the right problems.
3. A strong data infrastructure is critical
Data is at the heart of driving value from analytics. One of the things that came through in the study was a move toward shared data sources across both CPGs and retailers. The push toward building shared sources of truth across data assets is critical. If you don’t invest in the right data infrastructure, you risk creating dissonance in your own organization where you have teams leveraging different assets to answer similar problems and resulting with different answers. As you're maturing your analytics teams and building out your analytic strategy, it is important that you do it hand-in-hand with your data strategy. The value of your analytics is only as good as the data it sits on top of.
4. Drive experimentation and data to learn from
Reinforcement learning is a huge part of generative AI. The value of starting small is that you create really meaningful data that can be leveraged inside your system – and then drive context and learning for the future. Getting small solutions out quickly is helpful in learning what is most important to your users, customers, or business, and can act as a data source for future iterations. When branching into analytics, organizations should push for more experimentation and iterative solutions to drive learning. It gives you more to work with and a deeper understanding about the problem space that you're working in.
5. AI makes analytics more accessible
AI is reducing the barrier of entry for analytics in a lot of ways, creating democratization in who can do the analysis, and the skills required to do it. There's such a game of telephone in analytics. A stakeholder has a question that gets framed up by some layer of leadership that then gets passed to someone – an architect, a data scientist, an analyst – who leverages some set of tools to answer it. If AI can obfuscate the programming and technical acumen requirements to conduct analysis, anybody with a question can get an answer. I believe AI can compress the chain of analytic telephone and bring stakeholders closer to the source for answers, making the key more about a well-framed prompt and understanding of the problem than about programming.
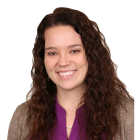
Visit our knowledge hub
See what you can learn from our latest posts.