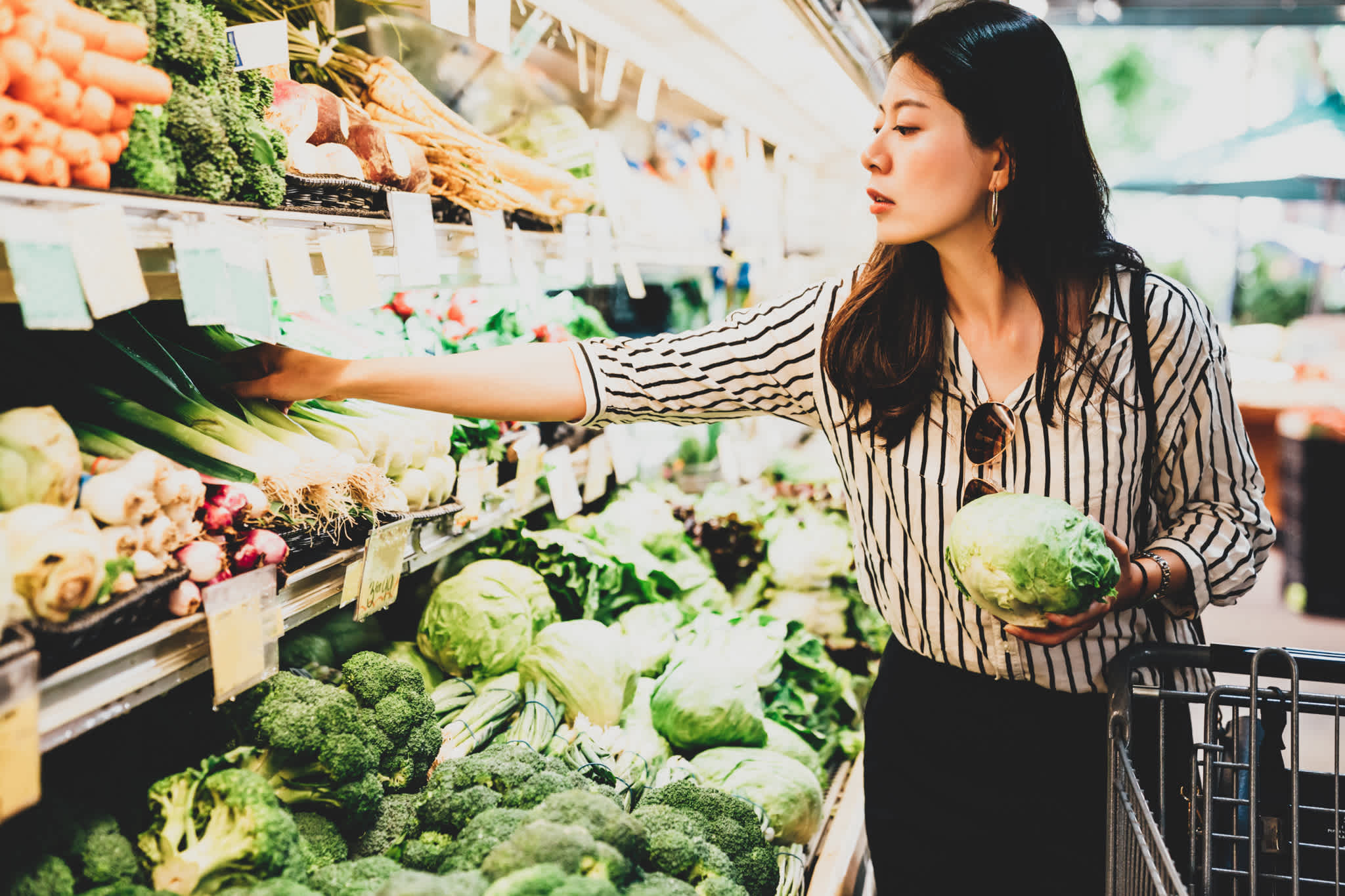
Go local: Get localized assortments right with data science
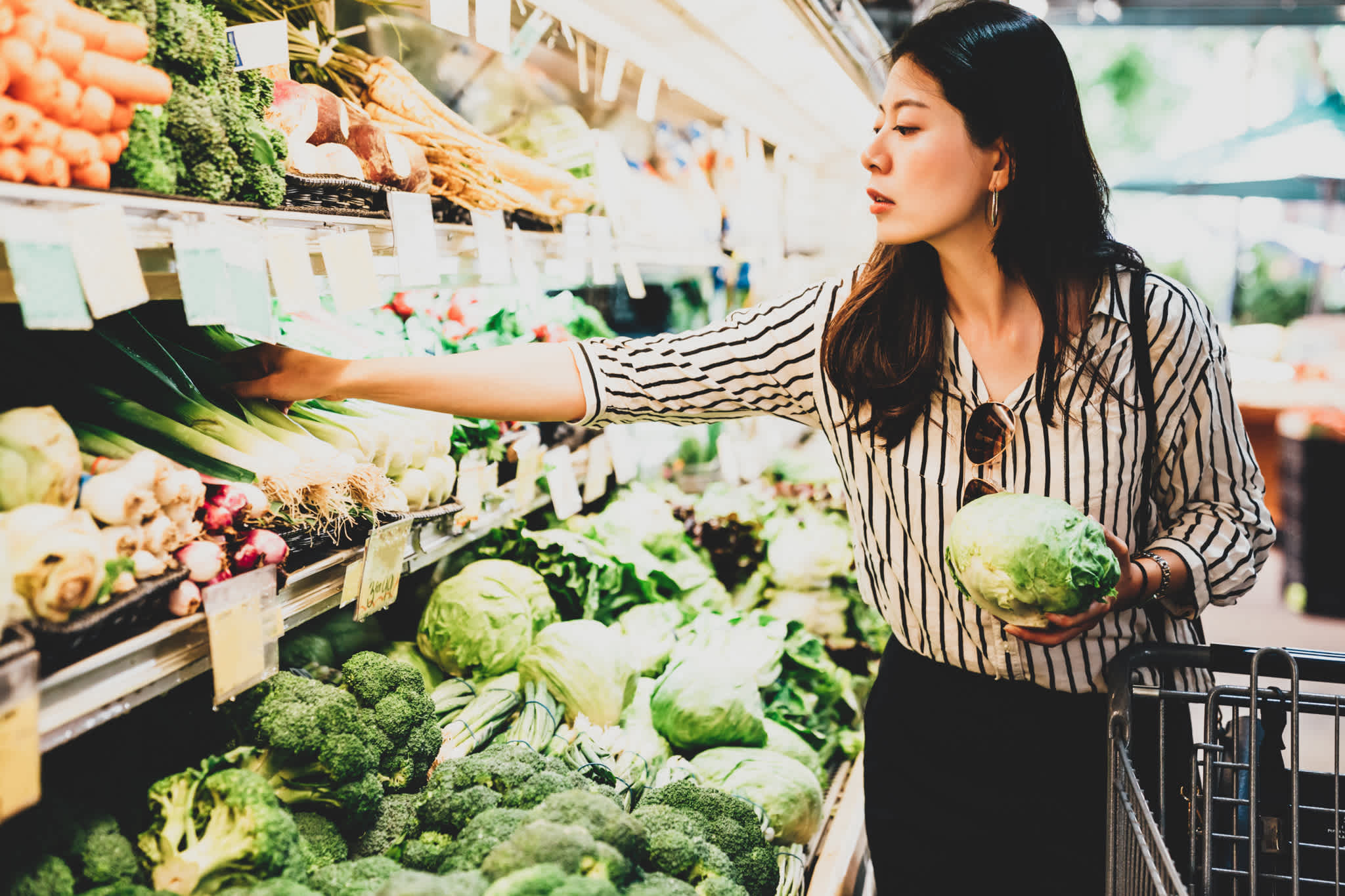
Generic, cookie-cutter retail concepts are out. Local is in. Consumer packaged goods (CPG) companies and retailers are increasingly taking a curated approach to their merchandise and stores, but scaling localized assortments requires an insight-rich strategy and close collaboration between brands and retailers. When CPGs and retailers work together to scale local assortments, they can better meet customer needs and build stronger customer relationships.
Why local
A growing number of retailers are taking a locally curated approach to merchandising and stores because today’s consumers expect personalization. They’re looking for items and experiences that meet their needs.
Also, different neighborhoods have different needs. A neighborhood with young families, for example, likely needs more diapers than a commercial area. To provide a meaningful customer experience, stores must serve the unique needs of the neighborhoods they call home.
Localized assortment as strategy
A localized assortment strategy uses insights from and about the neighborhood to help retailers determine the right product assortment to carry in each store. Rather than providing the same assortment for all stores, a localized assortment of goods increases customer relevancy and optimizes the store experience.
Successful localized store assortment strategies strike a balance between meeting customer needs, working within supply chain parameters and satisfying financial metrics. Data models can help achieve this goal by looking at very different aspects of an item’s performance across stores.
Our data models look at store purchases from every household related to that store. We track each item’s performance in terms of sales units, and we can also look at customer loyalty to that item by identifying its repeat purchase rate. This data helps retailers ensure they’re staying loyal to local households.
The science of selection
To develop a data-driven localized assortment strategy and then scale it, organizations must start with a prototype that includes an understanding of the problem, such as slow-moving inventory, and desired benefits. At 84.51°, our team of researchers brings together different sciences — from machine learning and optimization to statisticians — to look at this problem.
Once the problem is mapped out, we use simulation to examine the potential benefits of a localized assortment. Do we see a sales uplift? Do we serve the customer needs better given the current constraints? We do this simulation by proposing a localized strategy versus a current strategy, and we estimate the value by applying the new strategy.
The next step is a small-scale test. We work with category managers and stores to do AB tests, with A being the proposed way and B the current way, to see if we can verify that our simulation is correct. This stage involves testing details like store design, measurements and other statistics to measure the success of our method.
If we have both the theoretical and tested values, we’re ready for the scale phase, which brings in the engineering of how to integrate the solution with the store’s systems and develop the interface to bring the localized assortment to life. The whole process involves a broad, cross-functional team that includes data scientists, engineers and business stakeholders.
Finding the right balance
One of the challenges of scaling this science is that oftentimes, store items have complex interactions with each other. Some products are substitutable with each other, and some products are what we call synergistic with each other.
Even with localized preferences, you need to maintain a certain variety of different items to drive customers to the store. For example, carrying an assortment of chips in multiple flavors can boost the sales of each flavor when customers substitute one flavor for another. But if you only carry one flavor, people may not go to that store just for that single flavor, even if it’s a local or regional preference. Instead, they may go to a different store to purchase a different flavor altogether.
So, when you make assortment decisions, you need to consider: What if I remove this item? How many of those sales will transfer to another item? That’s where the science and machine learning, data and optimization model go to work to quantify the dynamics between assortment interactions and the value of those impacts. Those values will be the key metrics or decision factors needed to make the final assortment decision across categories and stores.
Working together to optimize local
It’s important for CPG companies to work together with retailers to optimize efficiency and customer product relevancy to deliver the right shopping experiences. On the category management side, our model helps stores manage assortment and provide feedback about how that assortment is working.
When we explain to CPGs what the assortment planning decision is and why we are making it, they can make better, more informed decisions about their promotional efforts. If we can tell them that we kept an item in the assortment because it’s good for a particular area, that’s feedback that can help the company revisit its marketing strategy with an eye on product relevancy and serving the neighborhood. Because when customers feel satisfied that the store assortment is both relevant and meaningful, it makes their lives easier, driving stronger relationships that build loyalty. Learn more about how 84.51° can help you understand and engage your customers along the path to purchase
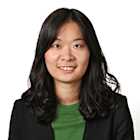
Visit our knowledge hub
See what you can learn from our latest posts.